Dr Umair Ul Hassan
Lecturer in Business Analytics & Society; Programme Director MSc Information Systems Management
J.E. Cairnes School of Business and Economics, University of Galway

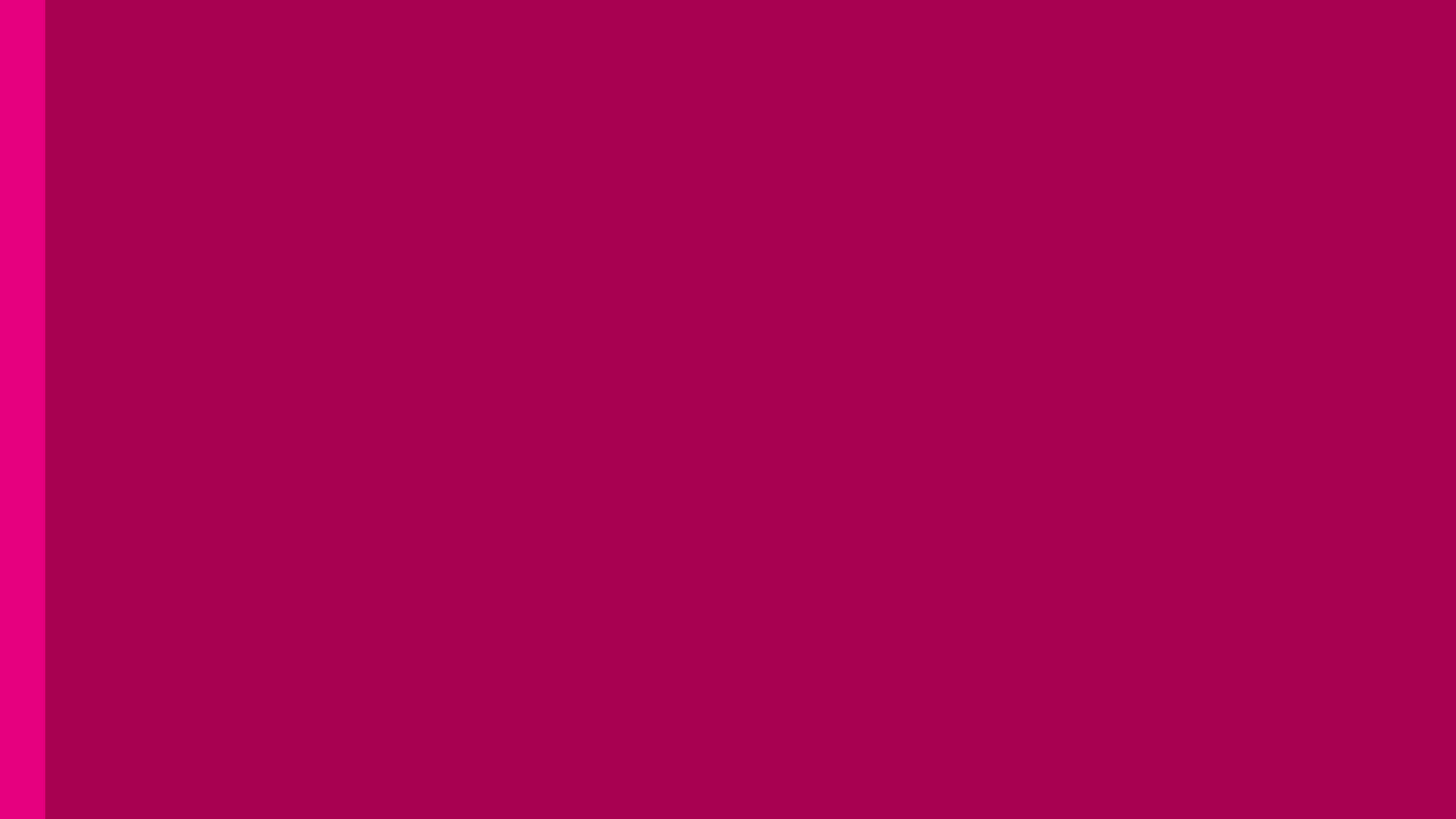
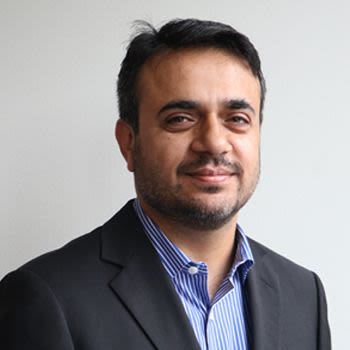
Umair's work focuses on design, development and the study of systems, using technologies such as artificial intelligence (AI), collective intelligence, database and information systems, to solve interesting business problems and to address societal challenges. Umair has been a Fellow in Lero and Principal Investigator for the SHAREPAIR and ENEPORTS projects with the Insight Centre for Data Analytics. He has also worked on research and innovation projects funded by the European Commission, Enterprise Ireland, and Science Foundation Ireland.
Umair's work contributes to these SDGs
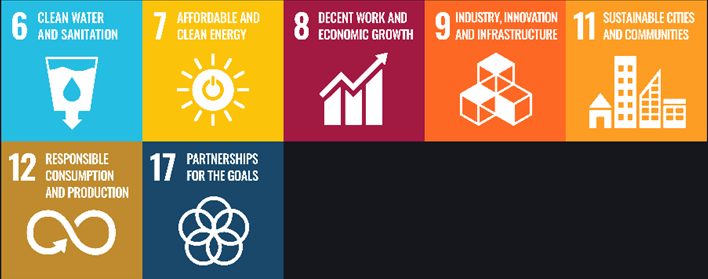
Umair is Principal Investigator on the EU-funded ENEPORTS - Decarbonization and Digitalization of Atlantic ports project. ENEPORTS examines how ports in the Atlantic Area are transforming towards digitalised green energy “laboratories”, preparing to supply clean electricity to ships and to their concessionaires (including blue/green energy manufacturers), and to facilitate testing and connection of renewable energy prototypes, taking advantage of local resources and proximity to the sea. ENEPORTS will examine how digitalisation and AI can contribute to manage energy flows and decarbonisation.
Target 7.A Promote access to research, technology and investments in clean energy
Target 12.5 Substantially reduce waste generation
Umair was Principal Investigator on the EU-funded SHAREPAIR Digital Support Infrastructure for Citizens in the Repair Economy project.
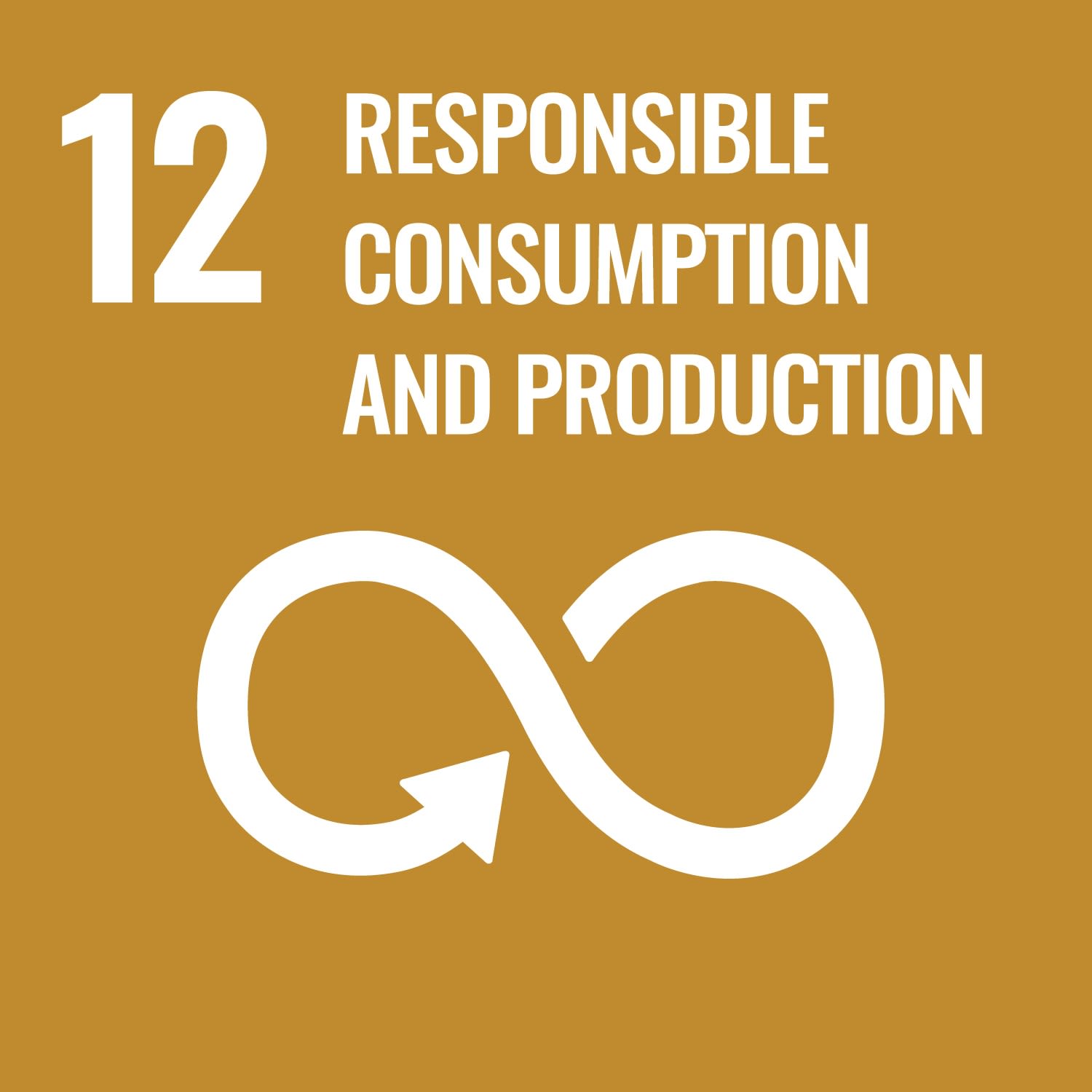
Teaching
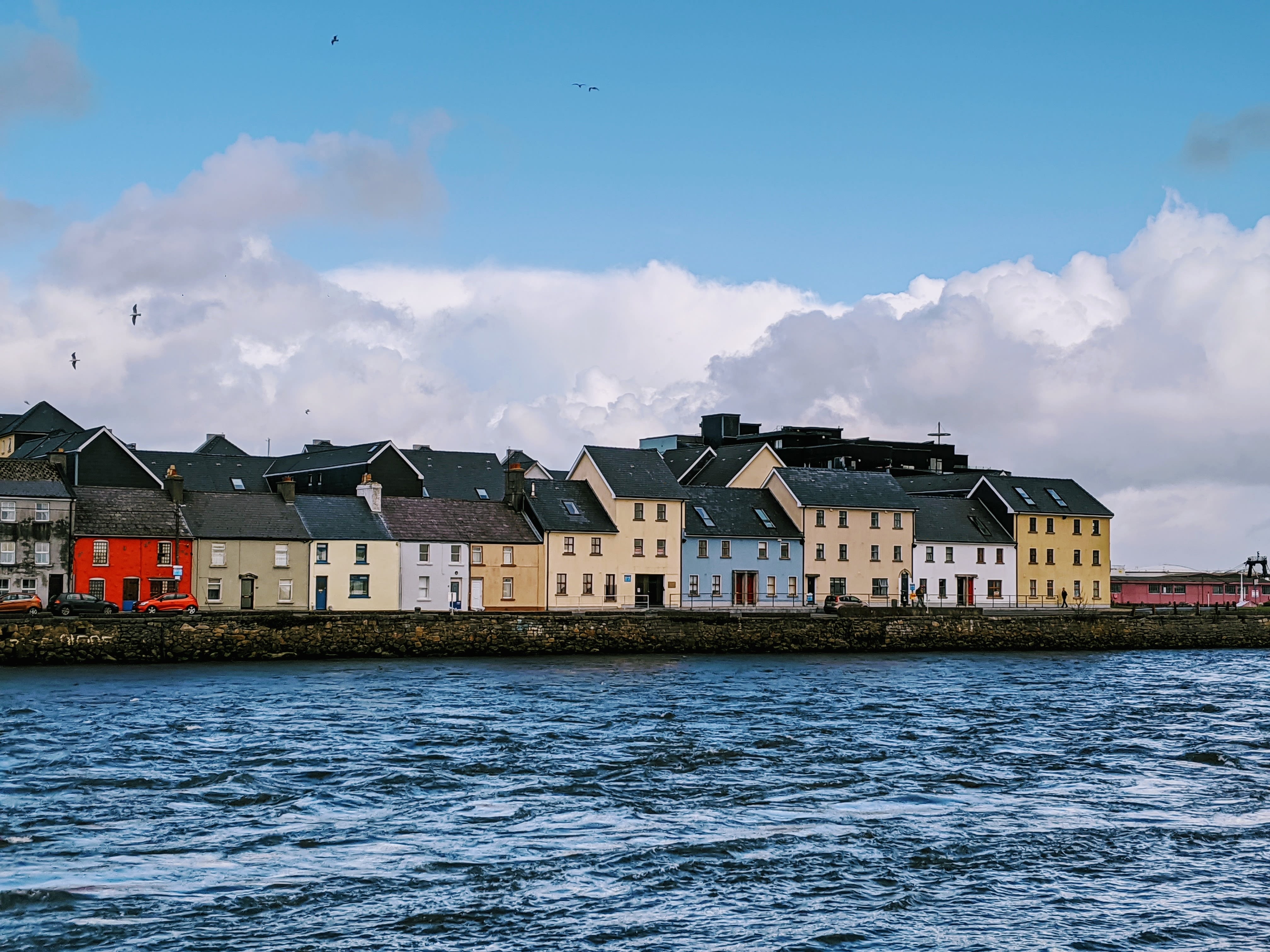
Umair teaches mainly technical modules such as networks and communication and advanced programming for data analytics. He endeavours where possible to give students the opportunity to use these skills to examine sustainability-oriented questions, using publicly available datasets to explore topics including sustainable purchasing, and product lifecycles.
Supporting Target: 17.18 Enhance availability of reliable data
Trust in AI Reflecting his extensive project and publication experience of artificial intelligence, Umair lectures on Trust and AI to law students at the School each year.
Supporting Target: 17.6 Knowledge sharing and cooperation for access to science, technology and innovation
Engagement
Umair's work has always has a strong public engagement focus, especially the ShareRepair project. His work has also featured a federated learning approach, describing an emerging area of data research with applications for manufacturing and Industry 4.0 in particular. Federated learning draws data from a multitude of IoT devices using a crowdsourcing approach. We find that the true potential of federated learning can only be realised if we have a dynamic and open ecosystem where devices, industrial units, machine manufacturers, non-governmental agencies and governmental entities can contribute toward learning for multiple tasks and objectives in a crowdsourced manner.
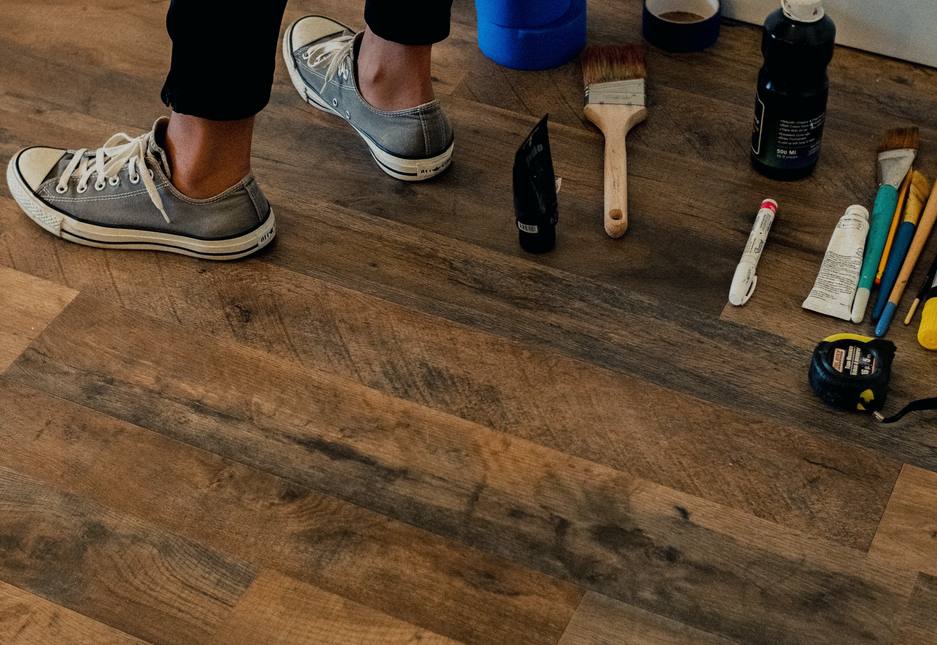
Direct impact SDG Targets
6.4 - Increase water-use efficiency and ensure freshwater supplies
6.5 - Implement integrated water resources management
7.3 - Double the improvement in energy efficiency
7.A - Promote access to research, technology and investments in clean energy
8.6 - Promote youth employment, education and training
9.5 - Enhance research and upgrade industrial technologies
9.C - Universal access to information and communications technology
11.2 - Affordable and sustainable transport systems
12.5 - Substantially reduce waste generation
17.6 - Knowledge sharing and cooperation for access to science, technology and innovation
17.18 - Enhance availability of reliable data
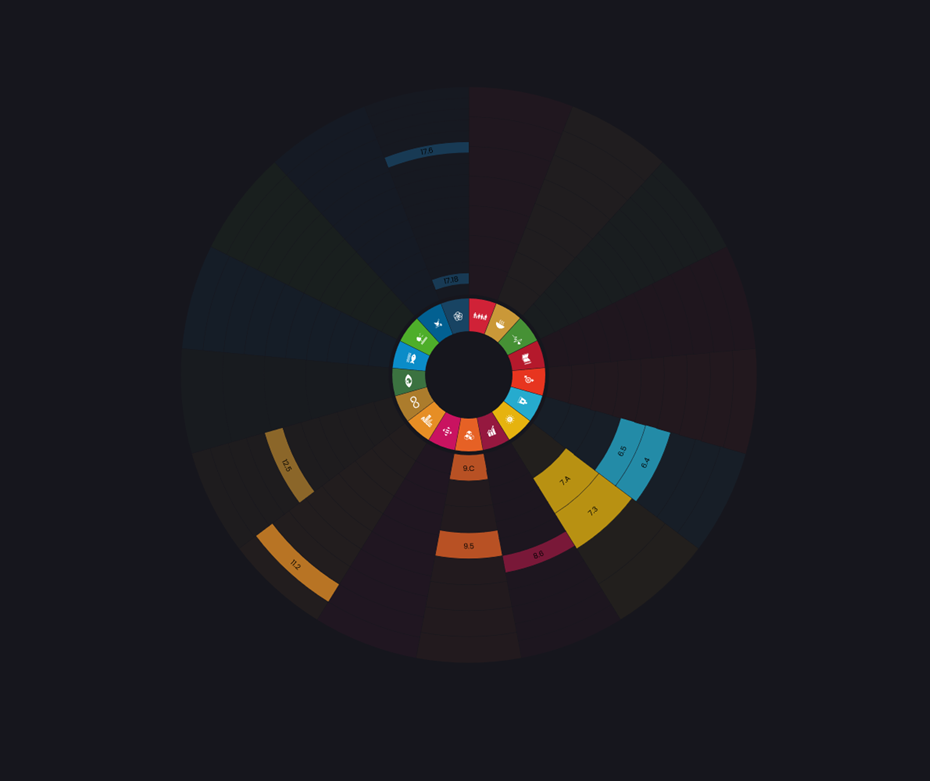
Umair's research has included but is not limited to Big Data Value Ecosystem (BDVe), Transforming Transport (TT), ICT for Water Resource Management (Waternomics), and the Big Data Roadmap and Cross-Disciplinary Community for Addressing Society Externalities (BYTE) project.
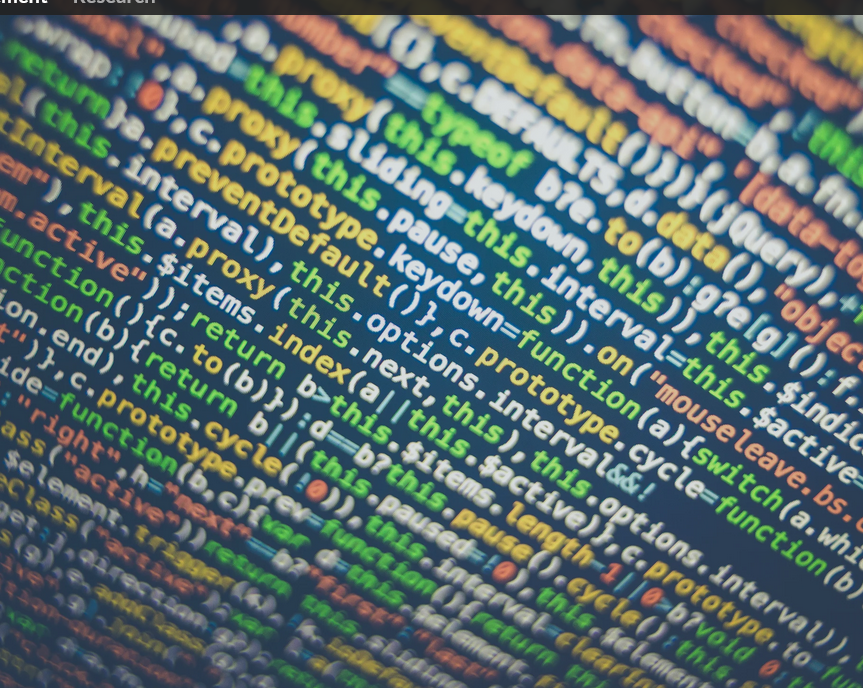
Research
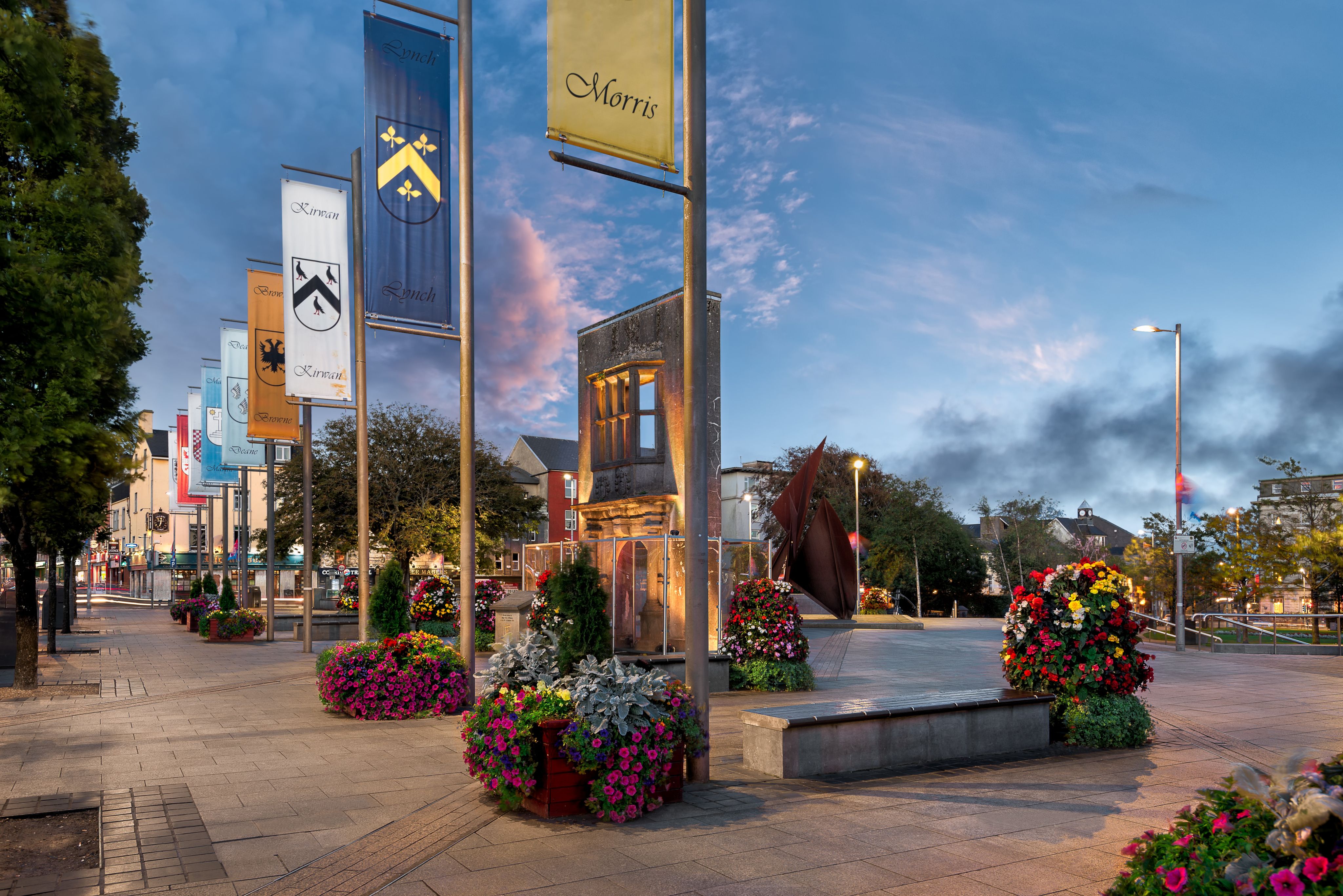
Featured Publications
Reference |
SDGs |
---|---|
ul Hassan, U., Curry, E. (2021). Stakeholder analysis of data ecosystems. The Elements of Big Data Value: Foundations of the Research and Innovation Ecosystem, 21-39. |
16 |
Mansoor, H., Ali, S., Alam, S. and 3 more (...) (2022). Impact of Missing Data Imputation on the Fairness and Accuracy of Graph Node Classifiers. Proceedings - 2022 IEEE International Conference on Big Data, Big Data 2022, 5988-5997. |
10 |
Ullah, I., Hassan, U. U., Ali, M. I. (2022). Multi-level Federated Learning for Industry 4.0 - A Crowdsourcing Approach. Procedia Computer Science, 217423-217435. |
9; 12; 13; 17 |
Curry, E., Derguech, W., Hasan, S. and 2 more (...) (2019). A Real-time Linked Dataspace for the Internet of Things: Enabling “Pay-As-You-Go” Data Management in Smart Environments. Future Generation Computer Systems, 90405-90422. |
6; 7 |
Curry, E., Hasan, S., Kouroupetroglou, C. and 3 more (...) (2018). Internet of Things Enhanced User Experience for Smart Water and Energy Management. IEEE Internet Computing, 22(1), 18-28. |
6; 7; 11 |
Curry, E., Derguech, W., Hasan, S. and 3 more (...) (2019). Building Internet of Things-Enabled Digital Twins and Intelligent Applications Using a Real-time Linked Dataspace. Real-time Linked Dataspaces: Enabling Data Ecosystems for Intelligent Systems, 255-270. |
6; 7; 11 |
Curry, E., Fabritius, W., Hasan, S. and 3 more (...) (2019). A Model for Internet of Things Enhanced User Experience in Smart Environments. Real-time Linked Dataspaces: Enabling Data Ecosystems for Intelligent Systems, 271-294. |
6; 7; 11 |